Home
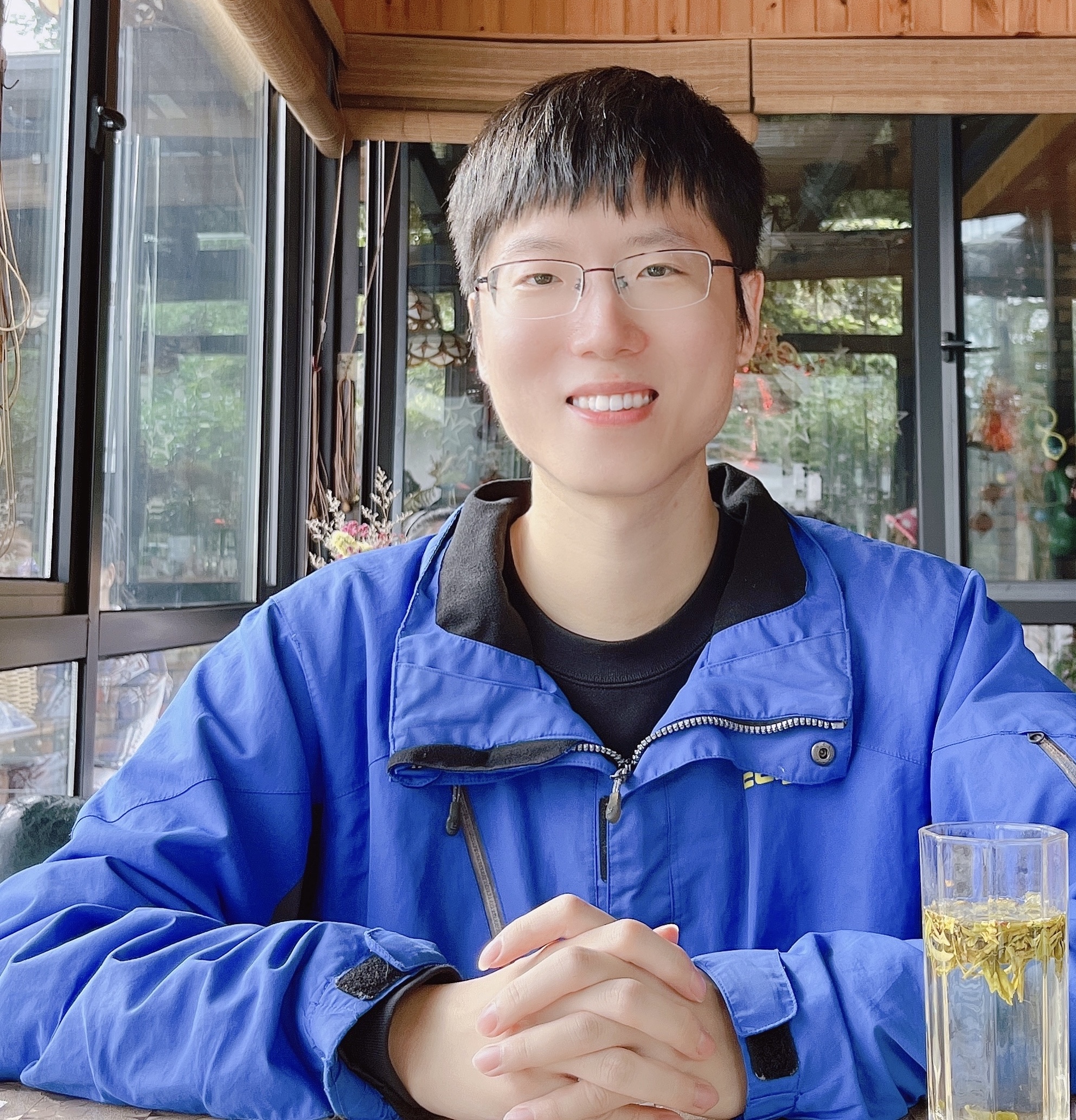 |
Chen Chen (谌晨)
I am a research scientist at Sony AI in the Privacy-Preserving Machine Learning team lead by Lingjuan Lyu.
Prior to that, I reveived my Ph.D. degree from Zhejiang University in 2022.
I am interested in trustworthy machine learning (privacy, security, etc). My current research focuses on federated learning and adversarial training.
[Google Scholar]
E-mail: chenchencc2021[at]gmail[dot]com
|
Education
Ph.D. in computer science and technology, Zhejiang University, China (Sep. 2017 - Dec. 2022)
Advisor: Professor Gang Chen
B.E. (honor) in computer science and technology, Chu Kochen Honors College (About 300 best students out of 6000 freshmen are chosen), Zhejiang University, China (Sep. 2013 - Jun. 2017)
Publications
(* indicates equal contributions)
How to Trace Latent Generative Model Generated Images without Artificial Watermark?
Zhenting Wang, Vikash Sehwag, Chen Chen, Lingjuan Lyu, Dimitris N. Metaxas, Shiqing Ma.
The Forty-First International Conference on Machine Learning (ICML 2024 ) .
COALA: A Practical and Vision-Centric Federated Learning Platform.
Weiming Zhuang, Jian Xu, Chen Chen, Jingtao Li, Lingjuan Lyu.
The Forty-First International Conference on Machine Learning (ICML 2024 ) .
A Simple Background Augmentation Method for Object Detection with Diffusion Model.
Yuhang Li, Xin Dong, Chen Chen, Weiming Zhuang, Lingjuan Lyu.
The European Conference on Computer Vision (ECCV 2024 ) .
CalFAT: Calibrated Federated Adversarial Training with Label Skewness.
Chen Chen, Yuchen Liu, Xingjun Ma, Lingjuan Lyu.
The Thirty-Sixth Conference on Neural Information Processing Systems (NeurIPS 2022).
International Workshop on Trustable, Verifiable and Auditable Federated Learning in Conjunction with AAAI 2022 (FL-AAAI 2022) Best Student Paper.
Online Partial Label Learning.
Haobo Wang, Yuzhou Qiang, Chen Chen, Weiwei Liu, Tianlei Hu, Zhao Li, Gang Chen.
Joint European Conference on Machine Learning and Knowledge Discovery in Databases (ECML 2020).
Experience
Research intern in Sony AI, Japan (Sep. 2021 — Dec. 2022)
Focused on privacy and robustness in federated learning. Lead a project that focuses on robustness (against adversarial attack) in federated learning. Specifically, the project considered federated adversarial training under the challenging non-IID setting.
Visiting Ph.D. in National University of Singapore, Singapore (Nov. 2019 — Nov. 2020)
Focused on robust federated learning, which can defend against Byzantine attacks and improve performance in federated learning.
Professional Service
Reviewer: ICML, NeurIPS, ICLR, AAAI, IJCAI, etc.
|